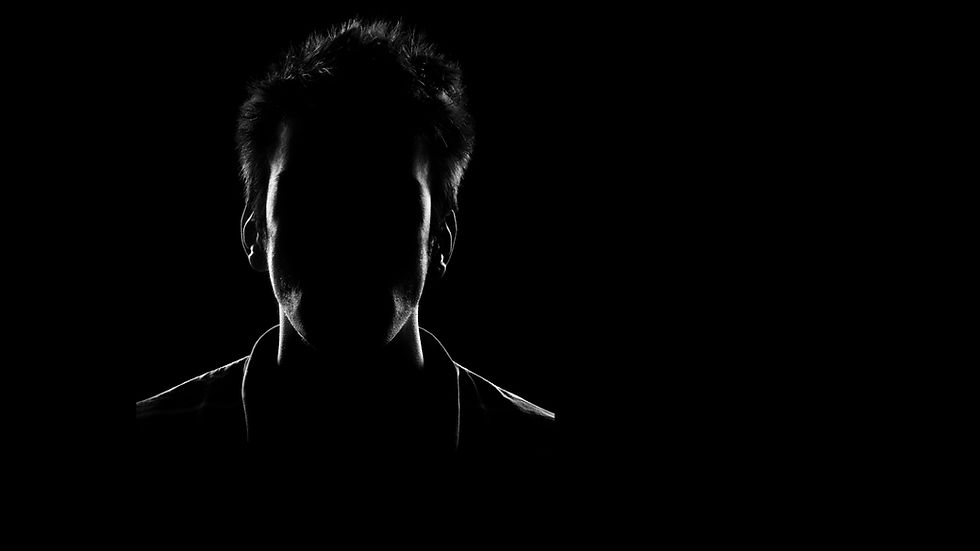
Photo by Quentin Arnaud on Behance
Complementing a deep understanding of the Data with Narrative and Visualization capabilities, Data Storytellers can help organizations translating from the language of data to the language of decision makers, becoming a key asset to accelerate value creation for the business.
Extracting insights from data has become a commonly accepted need by almost all organizations, but those who work with data know that insights aren’t written somewhere with the data or just there to be taken.
An emerging role in data science teams is that of the Data Storyteller who is responsible for extracting insights from the existing data and create compelling visualizations to clearly communicate the results achieved by the team.
In this article we'll explore why organizations who want to become more "data-driven" should understand how Data Storytellers can help them achieve this goal and present the skills required to master this important function.
Is this just a fancy name for BI Analysts or a new role I need to worry about?
If you are an executive or business leaders in any size company, chances are that you’re being overloaded with data, charts and dashboards you are asked on a daily basis to make key decisions on. Telling stories with data sounds a lot like what your BI team is doing already. After all this is why you have approved that budget for Analytics Visualization tools like, right? Well … sort of … Undoubtedly those are useful tools to run any business, but what happens when you have a team of data scientists looking at your data at a much deeper level with powerful techniques and start bringing to you new insights and recommendations that are perhaps not so apparent or intuitive? This is actually the core of the challenge: on the one hand you have hired these smart people to do exactly that — extracting insights from data at a much deeper level and helping the organization taking smarter decisions — on the other hand it is kind of hard to trust their conclusions because that stuff is too technical and a bit disjoint from the reality of your business. Stated this way, the situation described above — a lot more common these days than you might expect — is describing a communication challenge. A communication challenge between a technical data science team and the business team who are speaking very different languages and have a hard time making the expected progress. Now, you don’t solve this challenge having your business leaders learning to build neural networks or sending your data scientists to a Master in Business Administration program. You need a bridge between these two worlds and this is exactly where a Data Storyteller will help you the most. This is also why your BI team is of little help in this scenario: they are very good swimmers in the ocean of your data but they are not deep divers and are not technically equipped with the mathematical framework your data scientists have.
So, is this a new role then?
Not necessarily. In companies with a traditional “manufacturing production line” mindset, business functions are super specialized and segregated and a position is justified in the organization only if you can hire someone that act in that role all the times. In these companies we see managers either training Business Intelligence Analysts in data mining and machine learning techniques to allow them to “dive deeper” into the data or allow the Machine Learning Engineers and Data Scientists acquire the skills needed to better communicate their data stories. In companies further along in the AI Maturity journey that create value by having engaged cross-functional squads “owning the solution” and have richer interactions in an continuous and iterative fashion (let’s call them agile data squads), we see the emergency of a specific role of the Data Storyteller helping the team along throughout the project using data visualization techniques and taking the center stage when is time to present the results achieved to other teams or business stakeholders. Ultimately we see enough signals that Data Storytelling is becoming a key Capability required across the company, and enterprises serious about becoming “data-driven” are considering upskilling professionals in any company function — from Marketing Managers to Logistic team leader to Financial Managers and not relegated to the data analytics team.
Data Storytelling as decision support tool
Once the data science team has performed several machine learning experiments, evaluated a number of hypothesis and created a performing predictive model, they typically need to convince the business owners that their solution can help the organization achieve better results for the given use case and should move to production. Easier said than done.
The data scientists working in large corporations know that this is perhaps one of the steepest mountains they have to climb in their attempt to help their companies making better products and services. There is a long list of reasons that one can bring here to explain why so many models never get deployed, from regulatory compliance rules to limitations of internal legacy systems, but if we dig down most of the time we can trace these reasons back to a fundamental challenge: trust. And it goes beyond lack of trust between experienced business decision makers that “know what works and what doesn’t” in their business and the new smart kid in the block that is using some unorthodox black magic to suggest how some key decisions should be taken. It’s a lack of trust that morph into fear at a deeper level between a human and the “artificial intelligence” which appears to be very powerful and is challenging the decision maker’s very existence.
This topic is quite fascinating and opens the door for a growing and exciting field of Explainable AI (which we’ll dive into perhaps in another article). For the purpose of this article the point we want to bring home is that at this stage of any data science project, we are facing with two audiences: a technical team with some new smart solution based on their deep understanding of data and a business decision making team with a lot of domain knowledge that can at times translates into cognitive bias.
Data Storytelling bridges the gap between the analysis and the decisions in data-driven companies
A good data story can be a powerful bridge between the technical and business audiences which sometimes is all it takes to separate companies who take decisions fast and those who debate forever even minor points without moving one inch.
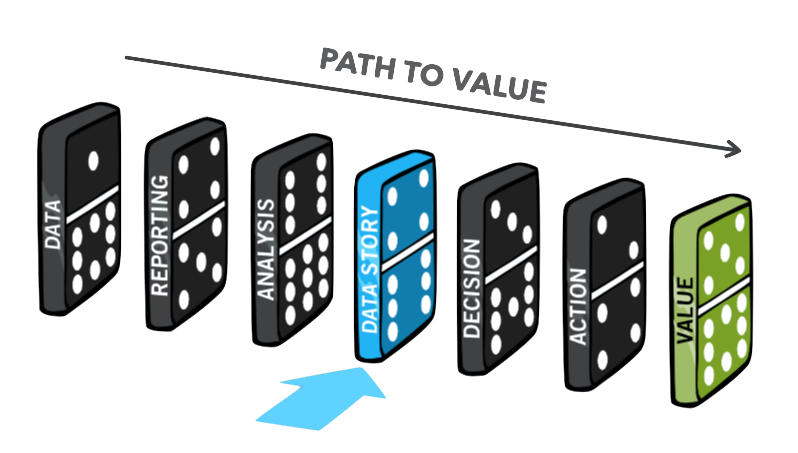
Created by Brent Dykes — Copyright 2015 Adobe Systems Incorporated
What skills should a Data Storyteller have?
A Data Storyteller is fundamentally a translator.
He/she needs to be able to dive deep into the data, extract key insights and effectively communicate them to the business. As such a number of skills across multiple domains are required, which makes this role difficult to fulfill and critical for data-driven organizations at the same time.
Data Storytellers should tell humans the story the data tells them
There are a lot of references available on the web about storytelling with data and a few good books as well, but perhaps the most complete framework to understand this role is the one created by Brent Dykes around 2015 at Adobe. Brent has recently codified what is needed to become a great Data Storyteller in his latest book “Effective Data Storytelling: how to drive change with data, narrative and visuals”, where he clearly articulate why the Data Storyteller should master a combination of skills at the intersection of these three domains:
Data
Narrative
Visuals
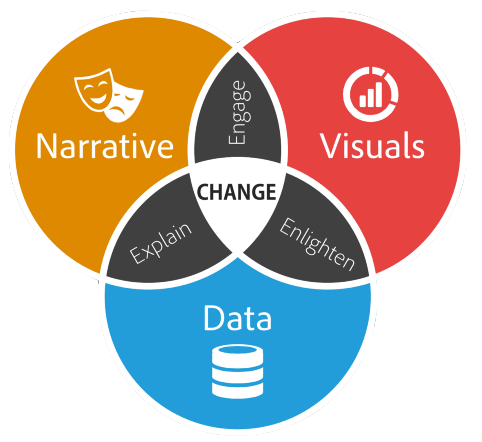
Data Storytelling Framework, Brent Dykes, “Effective Data Storytelling: how to drive change with data, narrative and visuals”, Wiley — December 2019
What’s nice about this framework is that incidentally explain why Data Storytelling is a stretch for the existing team: Data is definitely the domain of your data scientist team, Visualization is the domain currently owned by your business intelligence team while Narrative is perhaps the strength of your marketing team. You can probably place the people in your organization in a map like this through an internal assessment to find out who in your BI team is more data savvy or who in your marketing organization is more verse to create good data visualizations and define a professional growth path towards Data Storytelling for those who are interested to go down this road.
Let’s take a look at these three domains and see what each can bring to a powerful story told with data.
1. The Data foundation
A good Data Storyteller should understand the process of analyzing the data, which is the foundation for his/her story.
Exploratory Data Analysis
One of the initial activities in any data science projects is what’s called an Exploratory Data Analysis (EDA). This is perhaps one of the most critical step since is the first touchpoint the team has with the available data and very important information are extracted at this stage. The Exploratory aspect of this stage is very critical: when the team is in “discovery mode” people minds get inquisitive, all sorts of questions come naturally in their mind and — if equipped with the appropriate tools — a lot of hypothesis can be tested at this stage and some important answers can be obtained to start planning the data science experiments. The techniques applied at this stage allow the team to understand the quality of data, determine the statistical characteristics of the features in the dataset, identify if there is correlation among the available features and understand which features in the data have more predictive power.
Feature Engineering
Another critical task performed during a data science project is Feature Engineering — where data scientists apply transformations to the existing variables in the attempt to create new one with higher predicting power for the problem they are trying to solve.
Predictive Model Creation
The next stage is typically an experimentation phase where the team applies machine learning techniques for the creation of predictive models. This is where the data team dives a lot deeper into the data: a lot of assumptions are made at this stage, multiple hypothesis and techniques are tested and at each iteration the team learns more about the process they are analyzing through data science. Some times they emerge from the dive with golden insights and now they have to share with the rest of the world what they found and this is when having Data Storytelling capabilities pays off.
If the role of the Data Storyteller is not covered by the data scientists themselves, he/she would need to understand what is the process or techniques adopted to appreciate the insights extracted during the project development. He/she needs to be able to speak the language of the data scientists so to say. At the same time he/she needs to start to define what is the central insight of the story and what elements should be taken into the story to get the message across, speaking the language of the business.
2. Defining the Narrative
This is perhaps the most underdeveloped domain in today’s data projects, which is not surprising given the very technical nature of the data analysis and the fact that such analysis is often conducted by technical data people. Let’s take a look at some of the key skills a Data Storyteller needs to build a narrative for their data story.
Understand the psychology of decision makers
The first step to improve the way we communicate the results of your data science project to decision makers is to realize that decisions are not taken simply based on logic and data and there is always an emotional side of the decision making process — at least until humans are the ones taking decisions that is … This is why telling a story with your data is more effective than simply presenting some cold facts or numbers.
Understand your audience
This is a basic but often overlooked aspect. The way you present the conclusions reached during your data science project should be tailored to the people you tell the story to: who is your audience? what is their level of data fluency? what is your relationship with them? what are the desired outcomes?
The answer to these questions should impact your data storytelling strategy. For example:
If your audience is a Senior Data Scientist you would probably want structure your story in chronological order — starting with the experiments and assumptions taken going towards the results you obtained — you will have to be quantitative showing detailed metrics and KPIs and perhaps you would want to present showing your code on your Jupiter Notebook
if your audience is a board of executives you may want to present the conclusions first showing the recommended actions, you would probably show the high-level model impact and the decision model using perhaps a presentation.
Define the arc of the story
In order to guarantee your audience will follow you till the end is important to structure your data storytelling arc.
A typical structure has the following 4 parts:
Set-up: here is where you provide the context and present “the hook” — a key question you will provide an answer to with your document or presentation
Insights: here is where you share findings that reveal insights about the problem. A good Data Storyteller will order the insights in a way that builds up tension in their story leaving the most important point as last
Climax: here is where the most important and impactful insight is presented. Is where you want the decision makers really pay attention and start asking questions to make sure they truly understood this point.
Solution: at this point the Data Storyteller shares his/her recommendations and proposes next steps.
Define the heroes in your story
A good Data Storyteller is capable to determine which user segment matter the most to convey the messages he/she wants to communicate with his/her story. These can be easily extracted from the data used for the analysis and once defined, the Data Storyteller should give them a name and voice and present their journey. This is a good way to draw the audience attention to the story you are trying to tell.
Edit your story
Think about a 2 hours movie — that’s your entire data story. But not everyone has 2 hours to hear the full story and try to tell the full story in the 30 minutes the executives are giving you is like forcing them to watch your 2 hours movie at 4x Fast Forward. Sometimes you need a 2 minute trailer to sell your story. Knowing how to edit your story for the attention span of your audience — be it 5, 10, or 30 minutes — is an important skill a Data Storyteller needs to acquire.
Maintain the audience engaged
Defining the heroes is a good first step to maintain the audience engaged but it may not be enough. There are a number of techniques that can be used here from asking thought-provoking questions, to present the date in a way that your audience can easily relate to as well as using visualizations (the third pillar of Data Storytelling) in an engaging way.
These skills should be mastered regardless of how the story is delivered, whether is a live presentation or through a written document or an email.
3. Creating Visualizations
Visualization techniques can be very powerful to present information and insights available in the data. Because we are naturally attracted to visuals (and because learning how to use a new visualization library is easier for a data professional) often visualizations are created before any narrative for the story has been defined. This is also the reason why often visualizations are less impactful than they could be and sometimes they fall flat in front of the decision makers' eyes.
There is no lack of tools and libraries to easily create compelling visualizations but a Data Storytelling is less about fancy visuals and more about helping decision makers see the insights the team has extracted from the data. Below are a few aspects you should know when attempting to express your story with visuals.
Keep it simple
It is easy to get carried away because you have so much you want to share and when this happen your charts become difficult to digest and you can overload your audience. You want to convey a key message per chart or slide and strive for a minimalist approach: eliminate all the noise in your charts, i.e. keep an element in the visualization only if is adding valuable information and remove all the rest.
Learn the Grammar of Graphics
Visualization is a communication language on its own and as any other language it has its own structure and rules. The key here is select each element of the visualization not on the basis of some subjective aesthetic judgment but appropriately using the rules of the visualization language. Fortunately this is something you can learn since has been clearly codified. To understand what type of chart is more appropriate to convey your message, how to use geometric elements such as shapes and lines, how to encode information on specific dimensions, how to appropriately choose statistics and so forth, look no further than Leland Wilkinson's Grammar of Graphics, a seminal work in the space visualizations. If you want a quick look at these concepts, this article shows how to apply the key concepts.
Build Interactive Charts
Depending on your audience, sometimes it may be appropriate to build some interactivity into the visualization. Perhaps you need to show a key variable or result over time, perhaps you want your audience to explore the impact of a feature on the outcome of your model. Interactive dashboards and charts can help you convey more insights to audiences that have the time and interest to really go deeper into the nuances of your results. Here too there are a lot of great tools and libraries can help you achieve your goals, from Plotly to Bokeh, from Shiny Dashboards to D3.js to name a few of the popular ones these days.
No matter the technique used, make sure you use the visualization with purpose, engaging your audience on one side while making sure you bring your insights in the forefront.
Conclusion
In a world where companies are pursuing the objective of being more “data-driven”, the ability to extract insights from the enormous amount of data available and to take decisions based on these insights is a critical capability. Complementing a deep understanding of the Data with Visualization and Narrative capabilities, Data Storytellers can help organizations “translating” from the language of data to the language of decision makers becoming a key asset to accelerate value creation for the business.
Comments